Development of deep learning model for auto-detection of atmospheric gravity waves
HKO News Bulletin for the Aviation Community (40th Issue)
Atmospheric gravity waves (AGW) can trigger aircraft turbulence which is a major aviation hazard. AGW can be generated by various mechanisms such as airflow over mountains, by deep convection or by frontal systems. As AGW are characterised by their stripe-pattern, with advances in artificial intelligent in pattern recognition, use of satellite imageries for automatic detection of AGW is made possible.
The Hong Kong Observatory, in collaboration with NVIDIA, developed a deep learning model using the Faster Region-based Convolutional Neural Network (Faster RCNN) for auto-detection of AGW. The model was pre-trained with the Microsoft Common Objects in Context (COCO) dataset from Tensorflow Model Zoo. It is subsequently trained with Himawari-8 high-pass filtered water vapour geostationary satellite imageries with human-truthed AGW locations. It was then validated using another independent set of imageries. Preliminary results showed rather good model skill and an example is shown in Figure 1.
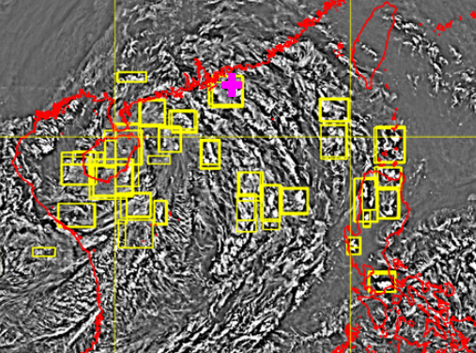
Figure 1: The high-pass filtered Himawari-8 geostationary satellite imagery at 06:40 UTC
on 8 October 2021 showed convection induced AGW locations captured by the deep
learning model (yellow boxes). The pink cross sign showed the location of pilot report
reporting severe turbulence.